Keras loss function understanding
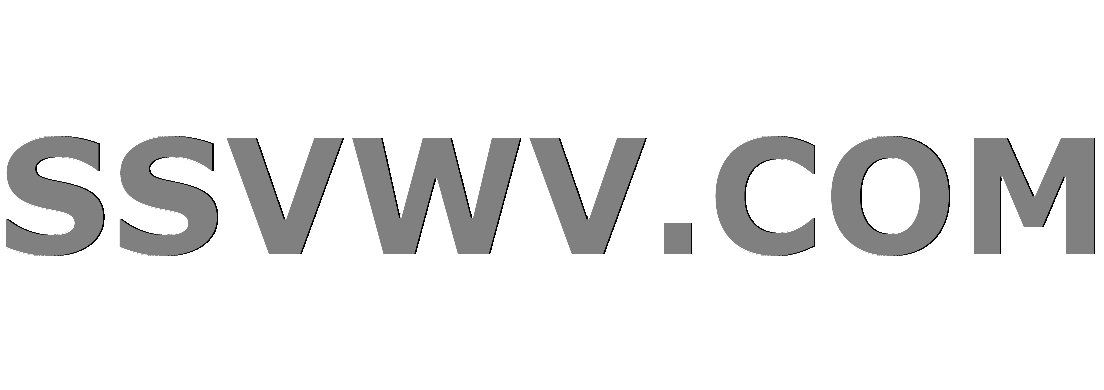
Multi tool use
up vote
0
down vote
favorite
In order to understand some callbacks of Keras better, I want to artificially create a nan
loss.
This is the function
def soft_dice_loss(y_true, y_pred):
from keras import backend as K
if K.eval(K.random_normal((1, 1), mean=2, stddev=2))[0][0] // 1 == 2.0:
# return nan
return K.exp(1.0) / K.exp(-10000000000.0) - K.exp(1.0) / K.exp(-10000000000.0)
epsilon = 1e-6
axes = tuple(range(1, len(y_pred.shape) - 1))
numerator = 2. * K.sum(y_pred * y_true, axes)
denominator = K.sum(K.square(y_pred) + K.square(y_true), axes)
return 1 - K.mean(numerator / (denominator + epsilon))
So normally, it calculates the dice loss, but from time to time it should randomly return a nan
. However, this does not seem to happen:
From time to time though, when I try to run the code, it stops right at the start (before the first epoch) with an error, saying that An operation has None for gradient. Please make sure that all of your ops have a gradient defined
Does that mean, that the the random function of Keras is just evaluated once and then always returns the same value?
If so, why is that and how can I create a loss function that returns nan
from time to time?
python tensorflow keras nan loss
add a comment |
up vote
0
down vote
favorite
In order to understand some callbacks of Keras better, I want to artificially create a nan
loss.
This is the function
def soft_dice_loss(y_true, y_pred):
from keras import backend as K
if K.eval(K.random_normal((1, 1), mean=2, stddev=2))[0][0] // 1 == 2.0:
# return nan
return K.exp(1.0) / K.exp(-10000000000.0) - K.exp(1.0) / K.exp(-10000000000.0)
epsilon = 1e-6
axes = tuple(range(1, len(y_pred.shape) - 1))
numerator = 2. * K.sum(y_pred * y_true, axes)
denominator = K.sum(K.square(y_pred) + K.square(y_true), axes)
return 1 - K.mean(numerator / (denominator + epsilon))
So normally, it calculates the dice loss, but from time to time it should randomly return a nan
. However, this does not seem to happen:
From time to time though, when I try to run the code, it stops right at the start (before the first epoch) with an error, saying that An operation has None for gradient. Please make sure that all of your ops have a gradient defined
Does that mean, that the the random function of Keras is just evaluated once and then always returns the same value?
If so, why is that and how can I create a loss function that returns nan
from time to time?
python tensorflow keras nan loss
add a comment |
up vote
0
down vote
favorite
up vote
0
down vote
favorite
In order to understand some callbacks of Keras better, I want to artificially create a nan
loss.
This is the function
def soft_dice_loss(y_true, y_pred):
from keras import backend as K
if K.eval(K.random_normal((1, 1), mean=2, stddev=2))[0][0] // 1 == 2.0:
# return nan
return K.exp(1.0) / K.exp(-10000000000.0) - K.exp(1.0) / K.exp(-10000000000.0)
epsilon = 1e-6
axes = tuple(range(1, len(y_pred.shape) - 1))
numerator = 2. * K.sum(y_pred * y_true, axes)
denominator = K.sum(K.square(y_pred) + K.square(y_true), axes)
return 1 - K.mean(numerator / (denominator + epsilon))
So normally, it calculates the dice loss, but from time to time it should randomly return a nan
. However, this does not seem to happen:
From time to time though, when I try to run the code, it stops right at the start (before the first epoch) with an error, saying that An operation has None for gradient. Please make sure that all of your ops have a gradient defined
Does that mean, that the the random function of Keras is just evaluated once and then always returns the same value?
If so, why is that and how can I create a loss function that returns nan
from time to time?
python tensorflow keras nan loss
In order to understand some callbacks of Keras better, I want to artificially create a nan
loss.
This is the function
def soft_dice_loss(y_true, y_pred):
from keras import backend as K
if K.eval(K.random_normal((1, 1), mean=2, stddev=2))[0][0] // 1 == 2.0:
# return nan
return K.exp(1.0) / K.exp(-10000000000.0) - K.exp(1.0) / K.exp(-10000000000.0)
epsilon = 1e-6
axes = tuple(range(1, len(y_pred.shape) - 1))
numerator = 2. * K.sum(y_pred * y_true, axes)
denominator = K.sum(K.square(y_pred) + K.square(y_true), axes)
return 1 - K.mean(numerator / (denominator + epsilon))
So normally, it calculates the dice loss, but from time to time it should randomly return a nan
. However, this does not seem to happen:
From time to time though, when I try to run the code, it stops right at the start (before the first epoch) with an error, saying that An operation has None for gradient. Please make sure that all of your ops have a gradient defined
Does that mean, that the the random function of Keras is just evaluated once and then always returns the same value?
If so, why is that and how can I create a loss function that returns nan
from time to time?
python tensorflow keras nan loss
python tensorflow keras nan loss
asked Nov 7 at 17:27
AljoSt
193212
193212
add a comment |
add a comment |
1 Answer
1
active
oldest
votes
up vote
0
down vote
Your first conditional statement is only evaluated once the loss function is defined (i.e. called; that is why Keras stops right at the start). Instead, you could use keras.backend.switch to integrate your conditional into the graph's logic. Your loss function could be something along the lines of:
import keras.backend as K
import numpy as np
def soft_dice_loss(y_true, y_pred):
epsilon = 1e-6
axes = tuple(range(1, len(y_pred.shape) - 1))
numerator = 2. * K.sum(y_pred * y_true, axes)
denominator = K.sum(K.square(y_pred) + K.square(y_true), axes)
loss = 1 - K.mean(numerator / (denominator + epsilon))
return K.switch(condition=K.random_normal((), mean=0, stddev=1) > 3,
then_expression=K.variable(np.nan),
else_expression=loss)
add a comment |
1 Answer
1
active
oldest
votes
1 Answer
1
active
oldest
votes
active
oldest
votes
active
oldest
votes
up vote
0
down vote
Your first conditional statement is only evaluated once the loss function is defined (i.e. called; that is why Keras stops right at the start). Instead, you could use keras.backend.switch to integrate your conditional into the graph's logic. Your loss function could be something along the lines of:
import keras.backend as K
import numpy as np
def soft_dice_loss(y_true, y_pred):
epsilon = 1e-6
axes = tuple(range(1, len(y_pred.shape) - 1))
numerator = 2. * K.sum(y_pred * y_true, axes)
denominator = K.sum(K.square(y_pred) + K.square(y_true), axes)
loss = 1 - K.mean(numerator / (denominator + epsilon))
return K.switch(condition=K.random_normal((), mean=0, stddev=1) > 3,
then_expression=K.variable(np.nan),
else_expression=loss)
add a comment |
up vote
0
down vote
Your first conditional statement is only evaluated once the loss function is defined (i.e. called; that is why Keras stops right at the start). Instead, you could use keras.backend.switch to integrate your conditional into the graph's logic. Your loss function could be something along the lines of:
import keras.backend as K
import numpy as np
def soft_dice_loss(y_true, y_pred):
epsilon = 1e-6
axes = tuple(range(1, len(y_pred.shape) - 1))
numerator = 2. * K.sum(y_pred * y_true, axes)
denominator = K.sum(K.square(y_pred) + K.square(y_true), axes)
loss = 1 - K.mean(numerator / (denominator + epsilon))
return K.switch(condition=K.random_normal((), mean=0, stddev=1) > 3,
then_expression=K.variable(np.nan),
else_expression=loss)
add a comment |
up vote
0
down vote
up vote
0
down vote
Your first conditional statement is only evaluated once the loss function is defined (i.e. called; that is why Keras stops right at the start). Instead, you could use keras.backend.switch to integrate your conditional into the graph's logic. Your loss function could be something along the lines of:
import keras.backend as K
import numpy as np
def soft_dice_loss(y_true, y_pred):
epsilon = 1e-6
axes = tuple(range(1, len(y_pred.shape) - 1))
numerator = 2. * K.sum(y_pred * y_true, axes)
denominator = K.sum(K.square(y_pred) + K.square(y_true), axes)
loss = 1 - K.mean(numerator / (denominator + epsilon))
return K.switch(condition=K.random_normal((), mean=0, stddev=1) > 3,
then_expression=K.variable(np.nan),
else_expression=loss)
Your first conditional statement is only evaluated once the loss function is defined (i.e. called; that is why Keras stops right at the start). Instead, you could use keras.backend.switch to integrate your conditional into the graph's logic. Your loss function could be something along the lines of:
import keras.backend as K
import numpy as np
def soft_dice_loss(y_true, y_pred):
epsilon = 1e-6
axes = tuple(range(1, len(y_pred.shape) - 1))
numerator = 2. * K.sum(y_pred * y_true, axes)
denominator = K.sum(K.square(y_pred) + K.square(y_true), axes)
loss = 1 - K.mean(numerator / (denominator + epsilon))
return K.switch(condition=K.random_normal((), mean=0, stddev=1) > 3,
then_expression=K.variable(np.nan),
else_expression=loss)
answered Nov 8 at 17:03
rvinas
3,8211328
3,8211328
add a comment |
add a comment |
Sign up or log in
StackExchange.ready(function () {
StackExchange.helpers.onClickDraftSave('#login-link');
});
Sign up using Google
Sign up using Facebook
Sign up using Email and Password
Post as a guest
Required, but never shown
StackExchange.ready(
function () {
StackExchange.openid.initPostLogin('.new-post-login', 'https%3a%2f%2fstackoverflow.com%2fquestions%2f53194704%2fkeras-loss-function-understanding%23new-answer', 'question_page');
}
);
Post as a guest
Required, but never shown
Sign up or log in
StackExchange.ready(function () {
StackExchange.helpers.onClickDraftSave('#login-link');
});
Sign up using Google
Sign up using Facebook
Sign up using Email and Password
Post as a guest
Required, but never shown
Sign up or log in
StackExchange.ready(function () {
StackExchange.helpers.onClickDraftSave('#login-link');
});
Sign up using Google
Sign up using Facebook
Sign up using Email and Password
Post as a guest
Required, but never shown
Sign up or log in
StackExchange.ready(function () {
StackExchange.helpers.onClickDraftSave('#login-link');
});
Sign up using Google
Sign up using Facebook
Sign up using Email and Password
Sign up using Google
Sign up using Facebook
Sign up using Email and Password
Post as a guest
Required, but never shown
Required, but never shown
Required, but never shown
Required, but never shown
Required, but never shown
Required, but never shown
Required, but never shown
Required, but never shown
Required, but never shown
uc2ieLChxGk