Enterprise ID assignment and record linkage
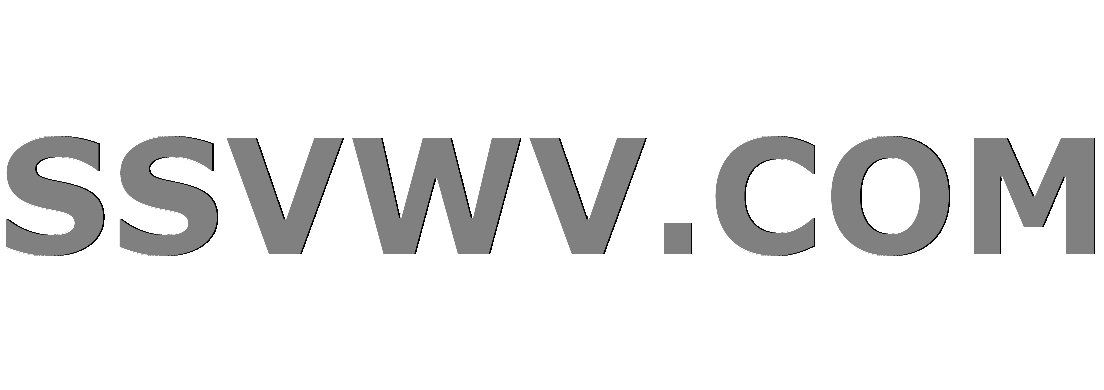
Multi tool use
up vote
0
down vote
favorite
I have a use case that’s solvable by ordinary methods but..
I want to find duplicates in customer data with low quality. Lack of standards, different order of names, punctuation, abbreviations, misspellings, etc
I need to be able to generate unique id’s for each person and cluster the records that refer to the same person under the same id.
There are roughly 1-2 billion records that would first need to be clustered.
After that, a scenario would be I get 100k to 20 million records and have to dedupe them against the larger dataset.
Conventionally, I could solve this via a normalization process, partitioning the records on something that drastically narrowed the search space and then manually figuring out some coded rules that caught most cases with a high amount of accuracy.
Easier said than done, but maybe doable. Maybe even the best way?
Or is there an appropriate use for deep learning here that can perform?
In my mind there are two primary challenges:
1) How to reliably determine if one record is a match for one other record, with lots of “fuzziness” built in
2) How to operate at such a volume of data and still scale
With things like Kubernetes, maybe I don’t need Spark? Or maybe Spark is better? Or something else?
machine-learning deep-learning bigdata scalability fuzzy-search
add a comment |
up vote
0
down vote
favorite
I have a use case that’s solvable by ordinary methods but..
I want to find duplicates in customer data with low quality. Lack of standards, different order of names, punctuation, abbreviations, misspellings, etc
I need to be able to generate unique id’s for each person and cluster the records that refer to the same person under the same id.
There are roughly 1-2 billion records that would first need to be clustered.
After that, a scenario would be I get 100k to 20 million records and have to dedupe them against the larger dataset.
Conventionally, I could solve this via a normalization process, partitioning the records on something that drastically narrowed the search space and then manually figuring out some coded rules that caught most cases with a high amount of accuracy.
Easier said than done, but maybe doable. Maybe even the best way?
Or is there an appropriate use for deep learning here that can perform?
In my mind there are two primary challenges:
1) How to reliably determine if one record is a match for one other record, with lots of “fuzziness” built in
2) How to operate at such a volume of data and still scale
With things like Kubernetes, maybe I don’t need Spark? Or maybe Spark is better? Or something else?
machine-learning deep-learning bigdata scalability fuzzy-search
add a comment |
up vote
0
down vote
favorite
up vote
0
down vote
favorite
I have a use case that’s solvable by ordinary methods but..
I want to find duplicates in customer data with low quality. Lack of standards, different order of names, punctuation, abbreviations, misspellings, etc
I need to be able to generate unique id’s for each person and cluster the records that refer to the same person under the same id.
There are roughly 1-2 billion records that would first need to be clustered.
After that, a scenario would be I get 100k to 20 million records and have to dedupe them against the larger dataset.
Conventionally, I could solve this via a normalization process, partitioning the records on something that drastically narrowed the search space and then manually figuring out some coded rules that caught most cases with a high amount of accuracy.
Easier said than done, but maybe doable. Maybe even the best way?
Or is there an appropriate use for deep learning here that can perform?
In my mind there are two primary challenges:
1) How to reliably determine if one record is a match for one other record, with lots of “fuzziness” built in
2) How to operate at such a volume of data and still scale
With things like Kubernetes, maybe I don’t need Spark? Or maybe Spark is better? Or something else?
machine-learning deep-learning bigdata scalability fuzzy-search
I have a use case that’s solvable by ordinary methods but..
I want to find duplicates in customer data with low quality. Lack of standards, different order of names, punctuation, abbreviations, misspellings, etc
I need to be able to generate unique id’s for each person and cluster the records that refer to the same person under the same id.
There are roughly 1-2 billion records that would first need to be clustered.
After that, a scenario would be I get 100k to 20 million records and have to dedupe them against the larger dataset.
Conventionally, I could solve this via a normalization process, partitioning the records on something that drastically narrowed the search space and then manually figuring out some coded rules that caught most cases with a high amount of accuracy.
Easier said than done, but maybe doable. Maybe even the best way?
Or is there an appropriate use for deep learning here that can perform?
In my mind there are two primary challenges:
1) How to reliably determine if one record is a match for one other record, with lots of “fuzziness” built in
2) How to operate at such a volume of data and still scale
With things like Kubernetes, maybe I don’t need Spark? Or maybe Spark is better? Or something else?
machine-learning deep-learning bigdata scalability fuzzy-search
machine-learning deep-learning bigdata scalability fuzzy-search
asked Nov 8 at 4:25


Tutan Ramen
647419
647419
add a comment |
add a comment |
active
oldest
votes
active
oldest
votes
active
oldest
votes
active
oldest
votes
active
oldest
votes
Thanks for contributing an answer to Stack Overflow!
- Please be sure to answer the question. Provide details and share your research!
But avoid …
- Asking for help, clarification, or responding to other answers.
- Making statements based on opinion; back them up with references or personal experience.
To learn more, see our tips on writing great answers.
Some of your past answers have not been well-received, and you're in danger of being blocked from answering.
Please pay close attention to the following guidance:
- Please be sure to answer the question. Provide details and share your research!
But avoid …
- Asking for help, clarification, or responding to other answers.
- Making statements based on opinion; back them up with references or personal experience.
To learn more, see our tips on writing great answers.
Sign up or log in
StackExchange.ready(function () {
StackExchange.helpers.onClickDraftSave('#login-link');
});
Sign up using Google
Sign up using Facebook
Sign up using Email and Password
Post as a guest
Required, but never shown
StackExchange.ready(
function () {
StackExchange.openid.initPostLogin('.new-post-login', 'https%3a%2f%2fstackoverflow.com%2fquestions%2f53201549%2fenterprise-id-assignment-and-record-linkage%23new-answer', 'question_page');
}
);
Post as a guest
Required, but never shown
Sign up or log in
StackExchange.ready(function () {
StackExchange.helpers.onClickDraftSave('#login-link');
});
Sign up using Google
Sign up using Facebook
Sign up using Email and Password
Post as a guest
Required, but never shown
Sign up or log in
StackExchange.ready(function () {
StackExchange.helpers.onClickDraftSave('#login-link');
});
Sign up using Google
Sign up using Facebook
Sign up using Email and Password
Post as a guest
Required, but never shown
Sign up or log in
StackExchange.ready(function () {
StackExchange.helpers.onClickDraftSave('#login-link');
});
Sign up using Google
Sign up using Facebook
Sign up using Email and Password
Sign up using Google
Sign up using Facebook
Sign up using Email and Password
Post as a guest
Required, but never shown
Required, but never shown
Required, but never shown
Required, but never shown
Required, but never shown
Required, but never shown
Required, but never shown
Required, but never shown
Required, but never shown
lHpM8RA8 TYsY sSk64ipNYx35xL9W9hUTICz,Br,7sV 2dxXir