How can I create custom loss function in Keras which is different for each sample
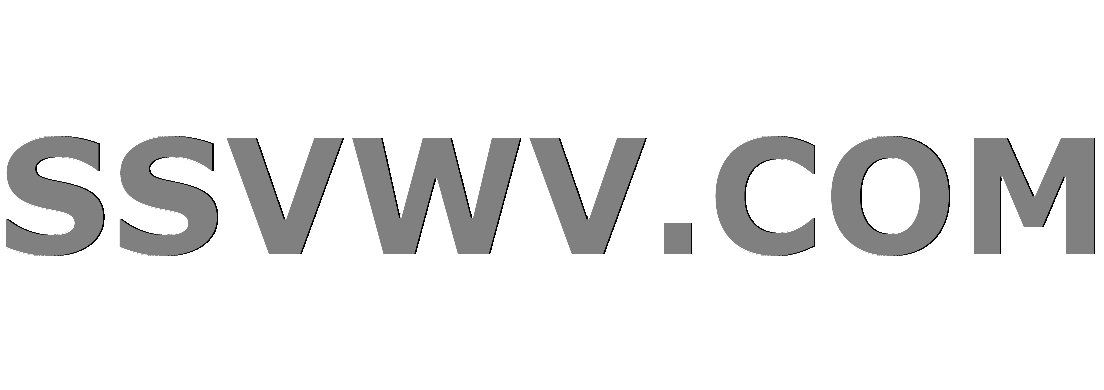
Multi tool use
.everyoneloves__top-leaderboard:empty,.everyoneloves__mid-leaderboard:empty,.everyoneloves__bot-mid-leaderboard:empty{ height:90px;width:728px;box-sizing:border-box;
}
I have e.g. 100 samples (100 outputs). I would like to write custom loss function with a "weight" for each sample:
(target[j] - prediction[j])**2 + f(j),
Where f is a custom numeric function (e.g. j**2
). How can I do this
Now I am only able to create "universal" loss function (without "weights"):
def customloss(target,prediction):
return (target - prediction)**2
The problem is I cannot get the index (j).
python keras loss
add a comment |
I have e.g. 100 samples (100 outputs). I would like to write custom loss function with a "weight" for each sample:
(target[j] - prediction[j])**2 + f(j),
Where f is a custom numeric function (e.g. j**2
). How can I do this
Now I am only able to create "universal" loss function (without "weights"):
def customloss(target,prediction):
return (target - prediction)**2
The problem is I cannot get the index (j).
python keras loss
Why dont you add an extra parameterweight
? A vector with the weight for each sample.
– Imanol Luengo
Nov 16 '16 at 18:55
The problem is AFAIK weights can only multiply results, so I would get sth like that: (target[j] - prediction[j])**2 * f(j) and I would like to add "weights" instead of multiply by them
– Filip Sokolowski
Nov 18 '16 at 15:56
add a comment |
I have e.g. 100 samples (100 outputs). I would like to write custom loss function with a "weight" for each sample:
(target[j] - prediction[j])**2 + f(j),
Where f is a custom numeric function (e.g. j**2
). How can I do this
Now I am only able to create "universal" loss function (without "weights"):
def customloss(target,prediction):
return (target - prediction)**2
The problem is I cannot get the index (j).
python keras loss
I have e.g. 100 samples (100 outputs). I would like to write custom loss function with a "weight" for each sample:
(target[j] - prediction[j])**2 + f(j),
Where f is a custom numeric function (e.g. j**2
). How can I do this
Now I am only able to create "universal" loss function (without "weights"):
def customloss(target,prediction):
return (target - prediction)**2
The problem is I cannot get the index (j).
python keras loss
python keras loss
edited Nov 16 '16 at 13:10


Div
4,41092647
4,41092647
asked Nov 16 '16 at 13:01
Filip SokolowskiFilip Sokolowski
61
61
Why dont you add an extra parameterweight
? A vector with the weight for each sample.
– Imanol Luengo
Nov 16 '16 at 18:55
The problem is AFAIK weights can only multiply results, so I would get sth like that: (target[j] - prediction[j])**2 * f(j) and I would like to add "weights" instead of multiply by them
– Filip Sokolowski
Nov 18 '16 at 15:56
add a comment |
Why dont you add an extra parameterweight
? A vector with the weight for each sample.
– Imanol Luengo
Nov 16 '16 at 18:55
The problem is AFAIK weights can only multiply results, so I would get sth like that: (target[j] - prediction[j])**2 * f(j) and I would like to add "weights" instead of multiply by them
– Filip Sokolowski
Nov 18 '16 at 15:56
Why dont you add an extra parameter
weight
? A vector with the weight for each sample.– Imanol Luengo
Nov 16 '16 at 18:55
Why dont you add an extra parameter
weight
? A vector with the weight for each sample.– Imanol Luengo
Nov 16 '16 at 18:55
The problem is AFAIK weights can only multiply results, so I would get sth like that: (target[j] - prediction[j])**2 * f(j) and I would like to add "weights" instead of multiply by them
– Filip Sokolowski
Nov 18 '16 at 15:56
The problem is AFAIK weights can only multiply results, so I would get sth like that: (target[j] - prediction[j])**2 * f(j) and I would like to add "weights" instead of multiply by them
– Filip Sokolowski
Nov 18 '16 at 15:56
add a comment |
1 Answer
1
active
oldest
votes
This might not be further relevant, but you can create a second network with an Input Layer. Towards that Input Layer you pass an Array that represents your weights.
Now wrap your model:
weight_layer = Input(shape=(None,dim))
m2 = Model(input=[m1.inputs,weight_layer],output=m1.outputs)
Since the output of a loss functions is also a Tensor you can add the weight_layer to your loss.
e.g.:
def customloss(y_true,y_pred):
return K.binary_crossentropy(y_true,y_pred) + weight_layer
m2.compile(optimizer='adam',loss=customloss,...)
add a comment |
Your Answer
StackExchange.ifUsing("editor", function () {
StackExchange.using("externalEditor", function () {
StackExchange.using("snippets", function () {
StackExchange.snippets.init();
});
});
}, "code-snippets");
StackExchange.ready(function() {
var channelOptions = {
tags: "".split(" "),
id: "1"
};
initTagRenderer("".split(" "), "".split(" "), channelOptions);
StackExchange.using("externalEditor", function() {
// Have to fire editor after snippets, if snippets enabled
if (StackExchange.settings.snippets.snippetsEnabled) {
StackExchange.using("snippets", function() {
createEditor();
});
}
else {
createEditor();
}
});
function createEditor() {
StackExchange.prepareEditor({
heartbeatType: 'answer',
autoActivateHeartbeat: false,
convertImagesToLinks: true,
noModals: true,
showLowRepImageUploadWarning: true,
reputationToPostImages: 10,
bindNavPrevention: true,
postfix: "",
imageUploader: {
brandingHtml: "Powered by u003ca class="icon-imgur-white" href="https://imgur.com/"u003eu003c/au003e",
contentPolicyHtml: "User contributions licensed under u003ca href="https://creativecommons.org/licenses/by-sa/3.0/"u003ecc by-sa 3.0 with attribution requiredu003c/au003e u003ca href="https://stackoverflow.com/legal/content-policy"u003e(content policy)u003c/au003e",
allowUrls: true
},
onDemand: true,
discardSelector: ".discard-answer"
,immediatelyShowMarkdownHelp:true
});
}
});
Sign up or log in
StackExchange.ready(function () {
StackExchange.helpers.onClickDraftSave('#login-link');
});
Sign up using Google
Sign up using Facebook
Sign up using Email and Password
Post as a guest
Required, but never shown
StackExchange.ready(
function () {
StackExchange.openid.initPostLogin('.new-post-login', 'https%3a%2f%2fstackoverflow.com%2fquestions%2f40632847%2fhow-can-i-create-custom-loss-function-in-keras-which-is-different-for-each-sampl%23new-answer', 'question_page');
}
);
Post as a guest
Required, but never shown
1 Answer
1
active
oldest
votes
1 Answer
1
active
oldest
votes
active
oldest
votes
active
oldest
votes
This might not be further relevant, but you can create a second network with an Input Layer. Towards that Input Layer you pass an Array that represents your weights.
Now wrap your model:
weight_layer = Input(shape=(None,dim))
m2 = Model(input=[m1.inputs,weight_layer],output=m1.outputs)
Since the output of a loss functions is also a Tensor you can add the weight_layer to your loss.
e.g.:
def customloss(y_true,y_pred):
return K.binary_crossentropy(y_true,y_pred) + weight_layer
m2.compile(optimizer='adam',loss=customloss,...)
add a comment |
This might not be further relevant, but you can create a second network with an Input Layer. Towards that Input Layer you pass an Array that represents your weights.
Now wrap your model:
weight_layer = Input(shape=(None,dim))
m2 = Model(input=[m1.inputs,weight_layer],output=m1.outputs)
Since the output of a loss functions is also a Tensor you can add the weight_layer to your loss.
e.g.:
def customloss(y_true,y_pred):
return K.binary_crossentropy(y_true,y_pred) + weight_layer
m2.compile(optimizer='adam',loss=customloss,...)
add a comment |
This might not be further relevant, but you can create a second network with an Input Layer. Towards that Input Layer you pass an Array that represents your weights.
Now wrap your model:
weight_layer = Input(shape=(None,dim))
m2 = Model(input=[m1.inputs,weight_layer],output=m1.outputs)
Since the output of a loss functions is also a Tensor you can add the weight_layer to your loss.
e.g.:
def customloss(y_true,y_pred):
return K.binary_crossentropy(y_true,y_pred) + weight_layer
m2.compile(optimizer='adam',loss=customloss,...)
This might not be further relevant, but you can create a second network with an Input Layer. Towards that Input Layer you pass an Array that represents your weights.
Now wrap your model:
weight_layer = Input(shape=(None,dim))
m2 = Model(input=[m1.inputs,weight_layer],output=m1.outputs)
Since the output of a loss functions is also a Tensor you can add the weight_layer to your loss.
e.g.:
def customloss(y_true,y_pred):
return K.binary_crossentropy(y_true,y_pred) + weight_layer
m2.compile(optimizer='adam',loss=customloss,...)
edited Feb 25 at 21:25
answered Nov 24 '18 at 17:22
mssmss
65
65
add a comment |
add a comment |
Thanks for contributing an answer to Stack Overflow!
- Please be sure to answer the question. Provide details and share your research!
But avoid …
- Asking for help, clarification, or responding to other answers.
- Making statements based on opinion; back them up with references or personal experience.
To learn more, see our tips on writing great answers.
Sign up or log in
StackExchange.ready(function () {
StackExchange.helpers.onClickDraftSave('#login-link');
});
Sign up using Google
Sign up using Facebook
Sign up using Email and Password
Post as a guest
Required, but never shown
StackExchange.ready(
function () {
StackExchange.openid.initPostLogin('.new-post-login', 'https%3a%2f%2fstackoverflow.com%2fquestions%2f40632847%2fhow-can-i-create-custom-loss-function-in-keras-which-is-different-for-each-sampl%23new-answer', 'question_page');
}
);
Post as a guest
Required, but never shown
Sign up or log in
StackExchange.ready(function () {
StackExchange.helpers.onClickDraftSave('#login-link');
});
Sign up using Google
Sign up using Facebook
Sign up using Email and Password
Post as a guest
Required, but never shown
Sign up or log in
StackExchange.ready(function () {
StackExchange.helpers.onClickDraftSave('#login-link');
});
Sign up using Google
Sign up using Facebook
Sign up using Email and Password
Post as a guest
Required, but never shown
Sign up or log in
StackExchange.ready(function () {
StackExchange.helpers.onClickDraftSave('#login-link');
});
Sign up using Google
Sign up using Facebook
Sign up using Email and Password
Sign up using Google
Sign up using Facebook
Sign up using Email and Password
Post as a guest
Required, but never shown
Required, but never shown
Required, but never shown
Required, but never shown
Required, but never shown
Required, but never shown
Required, but never shown
Required, but never shown
Required, but never shown
q,jOhjsY2Je,O9awODkhSWA,ALSx8rT6ApC Sst8xdyNjx4QErRVYApNCbTn,mBVE6E5zlAOCf9 gRLCi ur6B0P44OonEm,cv9lE,tVw dnf
Why dont you add an extra parameter
weight
? A vector with the weight for each sample.– Imanol Luengo
Nov 16 '16 at 18:55
The problem is AFAIK weights can only multiply results, so I would get sth like that: (target[j] - prediction[j])**2 * f(j) and I would like to add "weights" instead of multiply by them
– Filip Sokolowski
Nov 18 '16 at 15:56